When a time component is present, a dataset may be used to forecast values. This blog will discuss the different types of data that can be used to forecast values over a time period and the components of these data.
When performing time-series data analysis, we work with two types of data: Panel Data and Hierarchical Time Series Data. Before we can understand the Cross-Sectional Data, which is the type of data we have used up to now,
Types of Data
Cross-Sectional Data
This data has a fixed time period. If we’re performing Linear or Logistic regression on data that contains customer transaction details over the past two years, such data is known as a cross-sectional dataset.
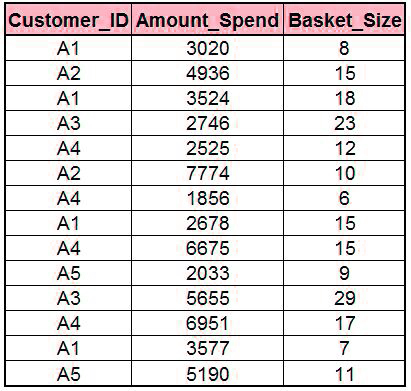
We can calculate various matrices, such as customer spending over the last two years, customer visits over the last three years, and average customer transaction value over three years. The time is fixed. We, therefore, calculate different metrics over time.
However, other types of data can be used to forecast values, such as Panel Data and Time Series.
Time series Data
In this example, the customer is the object that stays fixed. We have, for example, Customer A1 fixed. Additionally, we have a time component and other features.
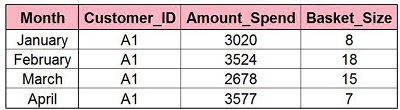
Comparing such data to Cross-Sectional data will help us understand the differences between them. While Cross-Sectional had the time component fixed while customers changed, Time Series Data has the same customer, but the time component is different. We have different data for the same customer across time.
Time Series data is particularly useful when the time period can be divided into equal intervals. If we have data for January and February and then November and December, then this data can’t be used. Also, data cannot be used if it contains missing periods. If we have month-wise data for a year but the data for the sixth month are missing, such data will not be of much use. The data should be equally spaced, i.e., If we have data up to the fourth month, but then we have weekly data, such data cannot be realized.
Panel Data
The Hierarchical Time Series Data is also known as Cross-Sectional data and Time Series data. If we have customer transaction data for multiple customers over the last three years, this data can be called Panel Data.
The same conditions apply to panel data as the Time Series data in order for it to be of any use.
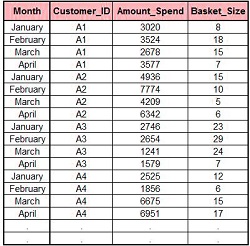
Usage of Time Series / Panel Data
These data types can be used to solve short-term and long-term business problems, such as forecasting stock prices or planning inventory. They are also used to forecast long-term values, such as forecasting GDP. This is where we need to consider many economic factors, such as income, population, prices, and other global factors.
Short, Medium & Long-term
Forecasting can be divided into three primary categories: short-, medium, and long-term.
Short Term – Scheduling Personnel and Transportation, Forecasting Demands, as part of the scheduling process.
Medium Term Calculate future resources required for the purchase of raw materials, hiring personnel, and purchasing machinery and equipment.
Long-Term Strategic Planning, taking into account opportunities, environmental factors, and internal resources
Examples
Here are a few examples of where such data is used regularly.
Example 1: Weather forecasting or Bullion forecasting, which falls under short-term forecasting.
Example 2: Stock prices forecast. These forecasts cannot be made long-term. They are done in the coming days, weeks, or months.
Example 3: An automobile manufacturer must purchase components from multiple sellers. It must forecast the demand for cars and keep inventory in line. This is medium-term forecasting.
Example 4: Cash management (Cash optimization). This could be done by determining how much money should be kept at each ATM. If the money is not used, it will cause a loss for the bank. ATMs that keep less money than they need will eventually run out of cash, which can lead to customer dissatisfaction as well as a loss for the bank.
Example 5. Forecasting Workforce Planning. This is short-medium-term forecasting. Customer support centers are an example of this. The customer calls can be different on different days or at different times of day (day or night). It is important to manage 50 employees. This forecasts the demand (call volume), which aids in optimizing the management of employees (optimization).
Example 6:Service Industries might need to plan for the recruitment process. If they project the projects coming their way, they can start the process.
Example 7: Growth of a country. This is called long-term forecasting.
Components Time Series Data
Although the Time Series data components have the same components as those found in Panel Data, it is much easier to understand these components in Time Series.
A time series data can be broken down into four components: Trend, Seasonality, and Cyclicity.
Trend
A trend is when data shows a trend. Although there might be fluctuations, it means that overall trends are increasing or decreasing. The trend line that we see below the orange line is the result of removing the seasonality component.
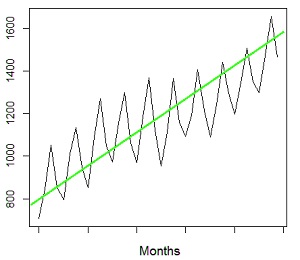
Seasonality
It refers to a pattern. It could be that sales slump in April, an increase in call volume on Saturdays and Sundays, or an abrupt rise in electricity demand in summers between 5-6 p.m. The trend is the peak or slump at different times. Example: Sales increase in December but decrease in March. We can see below that if we remove the trend from the above figure, we clearly understand the seasonality.
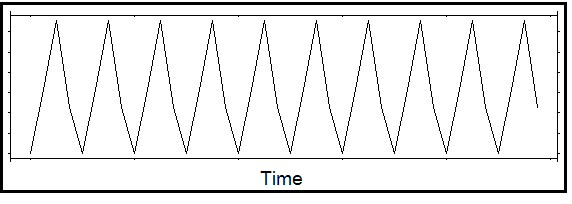
There are two types of seasonality
- In a day or week, i.e., Comparison between week and day
- Within a calendar year- Peak/slump at certain times of the year
Cyclicity
Data for many years can show cyclicity. A high number of sales each year due to an event, such as a spike in television sales due to the football world cup. Another example is a decrease in projects every four years due to elections that cause anxiety about the future policies of governments.
Irregularity
If the data does not show a trend, seasonality, or cyclicity
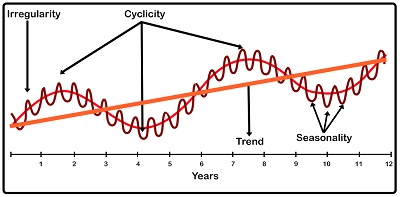
These four components are crucial in forecasting values using ARIMA and Smoothing techniques. As & when we have a better understanding of the types of data and the components of time-series data, we can explore the different techniques that can be used to forecast.